Max Noichl 1
This was a prize-winning entry into the Essay Competition on the Automation of Wisdom and Philosophy.
Summary
In this essay I will suggest a lower bound for the impact that artificial intelligence systems can have on the automation of philosophy. Specifically I will argue that skepticism is warranted about whether LLM-based systems similar to the best ones available right now will be able to independently produce philosophy at a level of quality and creativity that is interesting to us. But they are clearly already able to solve medium-complexity language tasks in a way that makes them useful to structure and consolidate the contemporary philosophical landscape, allowing for novel and interesting ways to orient ourselves in thinking.Introduction
The purpose of philosophical AI will be: To orient ourselves in thinking. This position is opposed to the view that the LLM-based artificial intelligence systems which are at this point foreseeable, will autonomously produce philosophy that is of a high enough quality and novelty to be interesting to us. In this essay I will briefly try to make this position plausible. I will then sketch the alternative direction in which I suspect the most impactful practical interaction of philosophy and AI will go and present a pilot study of what this may look like. Finally, I will argue that this direction can integrate well into contemporary philosophical practice and solve some previously unresolved desiderata.
Autonomous production of philosophy
The first idea that we might have when thinking about how artificial intelligence might serve to automate philosophy is that the AI system is going to philosophize for—instead —of us. And indeed the currently best publicly available systems seem to show some basic promise. They2 are able to recapitulate classic philosophical arguments and thought experiments with reasonable, although somewhat spotty, quality, and when vaguely prompted to opine on topics of philosophical impact they are also able to identify classical lines of argument.
But these abilities are very much in line with an understanding of LLMs that sees them largely as sophisticated mechanisms for the reproduction and adaptation of already present textual material, which would of course be in stark contrast to the capabilities that are arguably necessary for the production of truly novel and logically coherent philosophy, namely strong abstract reasoning capabilities.
Some grounds for scepticism
To me it seems like the capability profile of the language models we have seen so far is distinctly weird. They play chess, to some degree convincingly, although not well, but they are abysmal at tic-tac-toe. They can explain simulated annealing perfectly well, but can’t tell me reliably which countries in Europe start with a ‘Q’. Generally speaking, it seems to be hard to predict or intuit whether one of the current systems we have available will be good at a task without just trying it out. And of course, much harder still to predict what they will be good at in the future.
But I do believe that we have reasonable grounds for at least a certain amount of skepticism about whether really strong reasoning capabilities are around the corner. First, when trying to get LLMs to produce philosophical reasoning, it is common that they struggle to transfer argument schemes to novel contexts and to generalize them to domains that are not commonly used as examples in the literature. It also seems hard to keep them arguing a coherent point and to maintain truth and consistency through prolonged arguments. Finally, when simulating philosophical debates between multiple LLM agents, I have found them to be extremely stereotypical, repeating mostly stale commonplaces, and failing to come up with novel argumentative patterns—experiences which are in line with at least some lines of research that question current systems’ abstract reasoning capabilities.3
A lower bound
But publicly predicting that contemporary AI systems are unable to ever achieve this or that specific task has been a good method to force oneself to a public correction a few months later, or to stubbornly remain denying the obvious in increasingly ridiculous fashion.4
Therefore, instead of making any strong claims about the abilities of current or future AI systems, I suggest that the most productive way forward to consider the potential of automation of philosophy is to articulate what is reliably achievable with the systems that we have available now, and what plays into their current abilities and sidesteps their faults. As I have mentioned, a general formulation for the abilities of large language models will likely not be forthcoming. But a most uncontroversial provisional formulation instead might be something like: These systems excel at medium-complexity language tasks, which are similar to tasks solved in everyday language or well represented in public code bases. And of course, as computer systems, they excel in doing these tasks over and over again, many thousands of times.
The question we need to answer is thus, how philosophy might profit from a process of automation that plays into these precise strengths. Answering this question will provide us with a firm, unspeculative lower bound of what is possible in the automation of philosophy.
Making it more concrete
I have given some reason to think that the most likely short-term role for artificial intelligence within philosophy is not going to be through independently reasoning non-human intelligences that directly produce philosophy in a way that is on par, or superior to what we are able to do now. Rather, I argued, philosophy will be altered by the ability of artificial intelligence to integrate and structure large quantities of thought, which might drastically increase the cohesion of our collective philosophical enterprise. But this proposal might seem somewhat abstract. To give a more concrete idea of what I am thinking about, I have conducted a little pilot study.
For this pilot study, I have scraped the whole open-access bibliography of “philosophy of artificial intelligence” available on the PhilArchive.5 I have also searched for all articles containing ‘artificial intelligence’, ‘machine learning’, or ‘deep learning’ in the last 20 years among ten highly reputable Anglophone philosophy journals and integrated them into my dataset.
I then filtered out unusable texts—texts that were obviously not philosophy6, texts that were badly OCRed, and texts that were not in the English language, leaving me with a sample of 1,025 full-text articles.
In the first pass, I used GLiNER-large7, a flexible LLM-based Named Entity Recognition system, to search for entities that conformed to the working definition of “a philosophical theory or philosophical position, a view that attempts to explain or account for a particular problem in philosophy, or a named argument.”
This first pass extracted from each article a number of relatively low-quality but passable candidate positions, things like ‘naturalized moral psychology’, ‘naturalistic framework’, ‘moderate defense’, ‘human nature is bad’, ‘schools of thought’, ‘Confucian tradition’, etc.
In a second pass, these articles were fed to GPT-4o, which searched them for philosophical positions and parsed them into a structured data format, which contained a label for the position, a definition of the position that had to be drawn from the text, a number between -1 and 1 indicating whether the author was arguing in favor or against the position, with 0 indicating neutrality, and the exact passage at which this stance became apparent. The initial candidate positions extracted by GLiNER were used to identify potentially relevant candidate positions. These were then fed to GPT-4o to keep the naming in the dataset consistent.
At the end of this process, for our 1,025 papers, I had gathered a total of 6,059 distinct positions, which contained named positions and arguments like ‘functionalism’, ‘computationalism’, ‘the Chinese room argument’, ‘connectionism’, etc.
There are a number of potentially interesting analyses that are now possible on this dataset. But for this pilot project, I conducted an overview mapping, in which I combined two nearest-neighbor graphs, one that linked articles to articles with similar position profiles (articles arguing for, and denying the same things), and one based on semantic similarity, which was determined via embeddings produced through the all-mpnet-base-v28 language model. These graphs were combined, resulting in a new graph in which thematically similar texts are moved close together in the global picture, while groups of texts that are thematically similar but argue for different position profiles are locally split apart. This combined graph was then reweighted and laid out using uniform manifold approximation and projection in two dimensions.9
I then applied an hDBSCAN, a clustering algorithm, to this layout and marked the most relevant positions for each cluster on the two-dimensional layout. The results can be explored below:
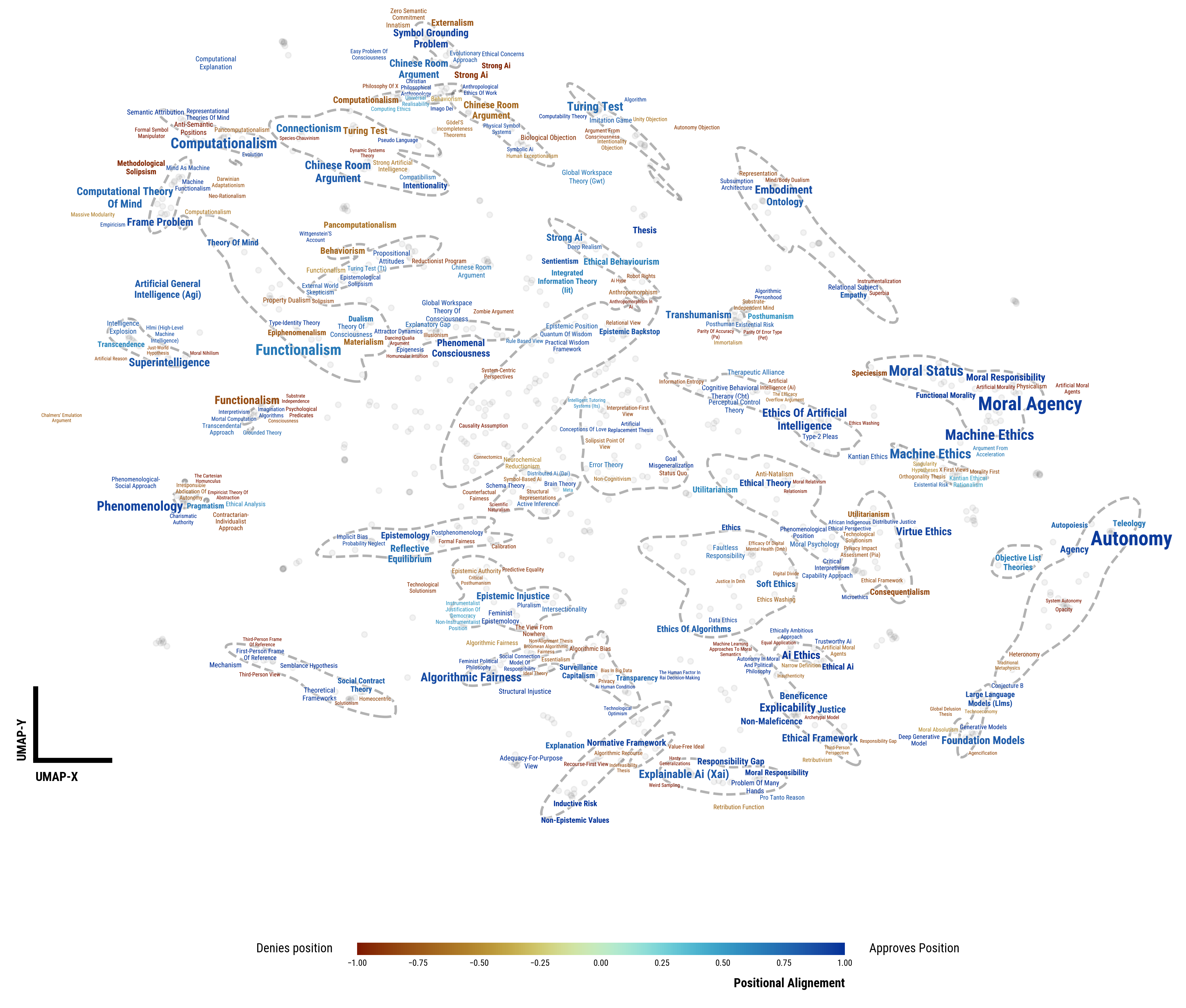
The clusters are marked with dashed lines, the grey points represent individual papers, and the positions are marked on top of them, with blue positions being those that are positively held by the authors in the cluster and red positions being denied.
We note that the map reproduces a sensible structure of the whole field with questions that relate artificial intelligence to the philosophy of mind towards the upper left, questions that relate to the moral status of artificial intelligence towards the center right, and questions about the societal impact of artificial intelligence and the connected ethical questions towards the bottom.
We also note that in quite a few instances we find clearly oppositional local structures, for example with clusters denying or appraising the Chinese room argument together with the appropriate associated stances on computationalism or universal realizability (middle-top). Similar things are true for functionalism (left-middle), as well as utilitarianism and virtue ethics (right-lower middle).
Philosophical relevance
I think that this pilot shows that, while quite a bit of additional work is evidently needed, contemporary LLM systems can reliably parse large amounts of philosophical text into structured representations that can be used to map out the argumentative landscapes. And while this is not automated philosophical reasoning, this is certainly not nothing.10 We commonly think about philosophy as a large, intractable net of interlinked arguments, where each single premise, if denied or accepted, has numerous implications for others, opening and closing paths to various positions—with philosophy arguably being the collective task of maintaining and refining this structure.
But this structure is never made explicit, and each philosopher, somewhat lonely, tends to produce philosophy in essays, which add to this whole structure only in a very local and convoluted fashion. The largest promise of automated philosophy as we can foresee it at this point, is thus to make this process explicit, to draw out the collective structure into the open, make it accessible, and, to borrow a phrase from Kant, to find a novel way to orient ourselves in thinking.11
I want to thank Christopher Zosh, Scott Page, Johannes Marx, John Miller, Melanie Mitchell, Arseny Moskvichev, and Robert Ward as well as my advisors Dominik Klein and Erik Stei for helpful discussions during the preparation of this essay. This project is part of my PhD at the department of theoretical philosophy at Utrecht University, and will be available soon in article form, alongside the code. Feel free to get in touch or learn more about my work via https://www.maxnoichl.eu/
Footnotes
Disclosure of AI usage: OpenAI’s GPT-4o was used as a coding assistant. OpenAI’s Whisper model was used to partially dictate this essay. OpenAI’s GPT-4o API was used for the presented analysis.↩︎
All the (informal) tests I have made in the process of writing this essay have been conducted on Anthropic’s Claude Opus model, OpenAI’s GPT-4o & GPT-4 Turbo, and Meta’s Llama 3 70B.↩︎
Lewis and Mitchell. Using Counterfactual Tasks to Evaluate the Generality of Analogical Reasoning in Large Language Models. 2024. arXiv: 2402.08955; Moskvichev, Odouard, and Mitchell. The ConceptARC Benchmark: Evaluating Understanding and Generalization in the ARC Domain. 2023. arXiv: 2305.07141↩︎
E. g. OpenAI’s o1-preview model was released a few weeks after the writing of this piece, and apparently achieved a marked increase in reasoning capabilities. When I gave it the final draft of this article to look over for typos, it did flag the “European countries with Q”-example I gave earlier as misleading, as there are, as o1-preview correctly noted, no such countries. I nonetheless currently belief that the arguments in this essay still hold.↩︎
Philosophy of Artificial Intelligence – Bibliography edited by Eric Dietrich – PhilArchive (accessed: 8.7.2024)↩︎
Many material machine-learning and computer-interaction articles somehow end up on PhilPapers, as well as a large collection of random things.↩︎
Zaratiana et al. *GLiNER: Generalist Model for Named Entity Recognition Using Bidirectional Transformer. 2023. arXiv: 2311.08526↩︎
Using the accessible implementation provided by Reimers and Gurevych. Sentence-BERT: Sentence Embeddings Using Siamese BERT-Networks. 2019. arXiv: 1908.10084↩︎
McInnes, Healy, and Melville. UMAP: Uniform Manifold Approximation and Projection for Dimension Reduction. 2018. arXiv: 1802.03426↩︎
And as a potential high-level interface between AI generated material and philosophers, it might also be crucial for the development of computer assisted philosophy, if the reasoning capabilities of the AI systems were to drastically improve.↩︎