In diabetic retinopathy, automated systems started out just below expert human level performance, and took around ten years to reach expert human level performance.
Details
Diabetic retinopathy is a complication of diabetes in which the back of the eye is damaged by high blood sugar levels.1 It is the most common cause of blindness among working-age adults.2 The disease is diagnosed by examining images of the back of the eye. The gold standard used for diabetic retinopathy diagnosis is typically some sort of pooling mechanism over several expert opinions. Thus, in the papers below, each time expert sensitivity/specificity (Se/Sp) is considered, it is the Se/Sp of individual experts graded against aggregate expert agreement.
As a rough benchmark for expert-level performance we’ll take the average Se/Sp of ophthalmologists from a few studies. Based on Google Brain’s work (detailed below), this paper 3, and this paper 4 , the average specificity of 14 opthamologists, which indicates expert human-level performance, is 95% and the average sensitivity is 82%.
As far as we can tell, 1996 is when the first algorithm automatically detecting diabetic retinopathy was developed. When compared to opthamologists’ ratings, the algorithm achieved 88.4% sensitivity and 83.5% specificity.
In late 2016 Google algorithms were on par with eight opthamologist diagnoses of diabetic retinopathy. See Figure 1.5 The high-sensitivity operating point (labelled on the graph) achieved 97.5/93.4 Se/Sp.
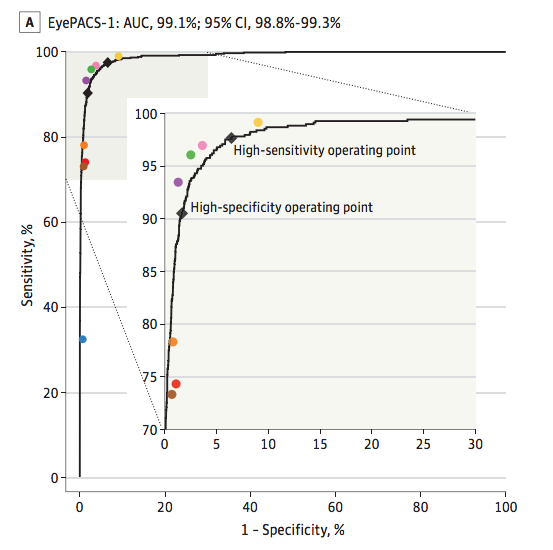
Many other papers were published in between 1996 and 2016. However, none of them achieved better than expert human-level performance on both specificity and sensitivity. For instance 86/77 Se/Sp was achieved in 2007, 97/59 in 2013, and 94/72 by another team in 2016. 6
Thus it took about ten years to go from just below expert human level performance to slightly superhuman performance.
Contributions
Aysja Johnson researched and wrote this page. Justis Mills and Katja Grace contributed feedback.
Footnotes
- See e.g. https://www.nhs.uk/conditions/diabetic-retinopathy/
- See https://nei.nih.gov/health/diabetic/retinopathy
- See Results section before adjudication and consensus https://www.ncbi.nlm.nih.gov/pubmed/23494039
- See Figure 3 https://www.ncbi.nlm.nih.gov/pmc/articles/PMC2911785/
- https://ai.googleblog.com/2016/11/deep-learning-for-detection-of-diabetic.html
- ‘Automated and semi-automated diabetic retinopathy evaluation has been previously studied by other groups. Abràmoff et al4 reported a sensitivity of 96.8% at a specificity of 59.4% for detecting referable diabetic retinopathy on the publicly available Messidor-2 data set.9Solanki et al12 reported a sensitivity of 93.8% at a specificity of 72.2% on the same data set. A study by Philip et al21 reported a sensitivity of 86.2% at a specificity of 76.8% for predicting disease vs no disease on their own data set of 14, 406 images.’ https://jamanetwork.com/journals/jama/fullarticle/2588763