Posted 13 July 2015
Participants
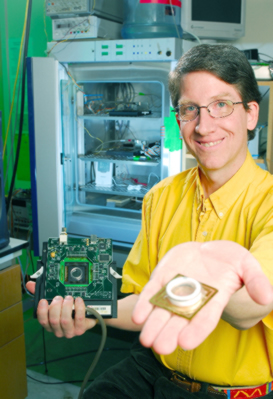
- Professor Steve Potter – Associate Professor, Laboratory of NeuroEngineering, Coulter Department of Biomedical Engineering, Georgia Institute of Technology
- Katja Grace – Machine Intelligence Research Institute (MIRI)
Note: These notes were compiled by MIRI and give an overview of the major points made by Professor Steve Potter.
Summary
Katja Grace spoke with Professor Steve Potter of Georgia Institute of Technology as part of AI Impacts’ investigation into the implications of neuroscience for artificial intelligence (AI). Conversation topics included how neuroscience now contributes to AI and how it might contribute in the future.
How has neuroscience helped AI in the past?
Professor Potter found it difficult to think of examples where neuroscience has helped with higher level ideas in AI. Some elements of cognitive science have been implemented in AI, but these may not be biologically based. He described two broad instances of neuroscience-inspired projects.
Subsumption architecture
Past work in AI has focused on disembodied computers with little work in robotics. Researchers now understand that AI does not need to be centralized; it can also take on physical form. Subsumption architecture is one way that robotics has advanced. This involves the coupling of sensory information to action selection. For example, Professor Rodney Brooks at MIT has developed robotic legs that respond to certain sensory signals. These legs also send messages to one another to control their movement. Professor Potter believes that this work could have been based on neuroscience, but it is not clear how much Professor Brooks was inspired by neuroscience while working on this project; the idea may have come to him independently.
Neuromorphic engineering
This type of engineering employs properties of biological nervous systems in neural system AI, such as perception and motor control. One aspect of brain function can be imitated with silicon chips through pulse-coding, where analog signals are sent and received in tiny pulses. An application for this is in camera development by mimicking pulse-coded signals between the brain and the retina.
How is neuroscience contributing to AI today?
Although neuroscience has not assisted AI development much in the past, Professor Potter has confidence that this intersection has considerable potential. This is because the brain works well in areas where AI falls short. For example, AI needs to improve how it works in real time in the real world. Self-driving cars may be improved through examining how a model organism, such as a bee, would respond to an analogous situation. Professor Potter believes it would be worthwhile research to record how humans use their brains while driving. Brain algorithms developed from this could be implemented into car design.
Current work at the intersection of neuroscience and AI include the following:
Artificial neural networks
Most researchers at the intersection of AI and neuroscience are examining artificial neural networks, and might describe their work as ‘neural simulations’. These networks are a family of statistical learning models that are inspired by biological neural networks. Hardware in this discipline includes neuromorphic chips, while software includes work in pattern recognition. This includes handwriting recognition and finding military tanks in aerial photographs. The translation of these networks into useful products for both hardware and software applications has been slow.
Hybrots
Professor Potter has helped develop hybrots, which are hybrid living tissue interfaced with robotic machines: robots controlled by neurons. Silent Barrage was an early hybrot that drew on paper attached to pillars. Video was taken of people viewing the Silent Barrage hybrots. This data was transmitted back to Prof. Potter’s lab, where it was used to trigger electrical stimulation in the living brain of the system. This was a petri dish interfaced to a culture of rat cortical neurons. This work is currently being expanded to include more types of hybrots. In one the control will be by living neurons, while the other will be controlled by a simulated neural network.
Meart (MultiElectrode Array Art) was an earlier hybrot. Controlled by a brain composed of rat neuron cells, it used robotic arms to draw on paper. It never progressed past the toddler stage of scribbling.
How is neuroscience likely to help AI in the future?
A particular line of research in neuroscience that is likely to help with AI is the concept of delays. Computer design is often optimized to reduce the amount of time between command and execution. The brain though may take milliseconds longer to respond. However delays in the brain were evolved to respond to the timing of the real world and are a useful part of the brain’s learning process.
Neuroscience probably also has potential to help AI in searching databases. It appears that the brain has methods for this that are completely unlike those used in computers, though we do not yet know what the brain’s methods are. One example given of the brain’s impressive abilities here is that Professor Potter can meet a new person and instantly be confident that he has never seen that person before.
How long will it take to duplicate human intelligence?
It will be hard to say when this has been achieved; success is happening at different rates for different applications. The future of neuroscience in AI will most likely involve taking elements of neuroscience and applying them to AI; it is unlikely that there will be a wait until we have a good understanding of the brain, then an export of that knowledge complete to AI.
Professor Potter greatly respects Ray Kurzweil, but does not think that he has an in depth knowledge of neuroscience. Professor Potter thinks the brain is much more complex than Kurzweil appears to believe, and that ‘duplicating’ human intelligence will take far longer than Kurzweil predicts. In Professor Potter’s consideration, it will take over a hundred years to develop a robot butler that can convince you that it is human.
Challenges to progress
Lack of collaboration
Neuroscience-inspired AI progress has been hampered because researchers across neuroscience and AI seldom collaborate with one another. This may be from disinterest or limited understanding of each other’s fields. Neuroscientists are not generally interested in the goal of creating human-level artificial intelligence. Professor Potter believes that of the roughly 30,000 people who attend the Society for Neuroscience, approximately 20 people want this. Most neuroscientists, for example, want to learn how something works instead of learning how it can be applied (e.g. learning how the auditory system works instead of developing a new hearing aid). If more people saw benefits in applying neuroscience to AI and in particular human-level AI, there would be greater progress. However, the scale is hard to predict. There is the potential for very much more rapid progress. For researchers to move their projects in this direction, the priorities of funding agencies would first have to move; these as these effectively dictate which projects move forward.
Funding
Funding for work at the intersection of neuroscience and AI may be hard to find. The National Institute of Health (NIH) funds only health-related work and has not funded AI projects. The National Science Foundation (NSF) may not think the work fits its requirement of being basic science research; it may be too applied. NSF though, is more open-minded to funding research on AI than NIH is. The military is also interested in AI research. Outside (of )the U.S., the European Union (EU) funds cross-disciplinary work in neuroscience and AI.
National Science Foundation (NSF) funding
NSF had a call for radical proposals, from which Professor Potter received a four-year-long grant to apply neuroscience to electrical grid systems. Collaborators included a power engineer and people studying neural networks. The group was interested in addressing the U.S.’s large and uneven power supply and usage. The electrical grid has become increasingly difficult to control because of geographically varying differences in input and output.
Professor Potter believes that if people in neuroscience, AI, neural networks, and computer design talked more, this would bring progress. However, there were some challenges with this collaborative electrical grid systems project that need to be addressed. For example, the researchers needed to spend considerable time educating one another about their respective fields. It was also difficult to communicate with collaborators across the country; NSF paid for only one meeting per year, and the nuances of in-person interaction seem important for bringing together such diverse groups of people and reaping the benefits of their creative communication.
Other people working in this field
- Henry Markram – Professor, École Polytechnique Fédérale de Lausanne, Laboratory of Neural Microcircuitry. Using EU funding, he creates realistic computer models of the brain, one piece at a time.
- Rodney Douglas – Professor Emeritus, University of Zurich, Institute of Neuroinformatics. He is a neuromorphic engineer who worked on emulated brain function.
- Carver Mead – Gordon and Betty Moore Professor of Engineering and Applied Science Emeritus, California Institute of Technology. He was a founding father of neuromorphic engineering.
- Rodney Brooks – Panasonic Professor of Robotics Emeritus, Massachusetts Institute of Technology (MIT). He was a pioneer in studying distributed intelligence and developed subsumption architecture.
- Andy Clark – Professor of Logic and Metaphysics, University of Edinburgh. He does work on embodiment, artificial intelligence, and philosophy.
- Jose Carmena – Associate Professor of Electrical Engineering and Neuroscience, University of California-Berkeley. Co-Director of the Center of Neural Engineering and Prostheses, University of California-Berkeley, University of California-San Francisco. He has researched the impact of electrical stimulation on sensorimotor learning and control in rats.
- Guy Ben-Ary – Manager, University of Western Australia, CELLCentral in the School of Anatomy and Human Biology. He is an artist and researcher who uses biologically related technology in his work. He worked in collaboration with Professor Potter on Silent Barrage.
- Wolfgang Maass – Professor of Computer Science, Graz University of Technology. He is doing research on artificial neural networks.
- Thad Starner – Assistant Professor, Georgia Institute of Technology, College of Computing. He applies biological concepts into developing wearable computing devices.
- Jennifer Hasler – Professor, Georgia Institute of Technology, Bioengineering and Electronic Design and Applications. She has studied neuromorphic hardware.
As the recent alphago vs lee sedol match has shown what the brain does is relatively simple.
What is complex and so spectacular is that it can do it so efficiently.
Creating a human 101
1. sensors – there are many artificial sensors available today that are significantly better than the human alternatives – from vision, to hearing, balance, chemical sensing, olfactory etc
2. processors power – cpu/gpu power for any given task is negligible – e.g. a 1 teraflop gpu can navigate a self-driving car better than most humans – has anyone asked why we need so much brain power if most tasks can be simulated with relatively low cpu/gpu requirements?
3. actuators for mimicking human motion are available
4. power source – many relatively efficient power sources are available (exclude side effects e.g. nuclear waste, CO2 emissions)
5. putting it all together and it’s possible to build a “humanoid android” ~ 3-4x the size of a human which can surpass
most humans in most activities – BUT
(i) it is still very inefficient
(ii) wont be accepted by society
Once costs of computing approach human levels costs (~ 15-30 years) AND we can package this in human-like form (available now) we are half way to creating a synthetic society.
The other half pertains to how we transfer human consciousness into these immortal bodies – NO IDEA.
At present Kurzweil’s approach of collating one’s life story and feeding it into a computer is one approach of
retaining one’s memories but how that integrates with a person’s personality is an area for research which
will come to the for only once the humanoid android body is created.