The cheapest hardware prices (for single precision FLOPS/$) appear to be falling by around an order of magnitude every 10-16 years. This rate is slower than the trend of FLOPS/$ observed over the past quarter century, which was an order of magnitude every 4 years. There is no particular sign of slowing between 2011 and 2017.
Support
Background
Computing power available per dollar has increased fairly evenly by a factor of ten roughly every four years in the last quarter of a century (a phenomenon sometimes called ‘price-performance Moore’s Law‘). Because this trend is important and regular, it is useful in predictions. For instance, it is often used to determine when the hardware for an AI mind might become cheap. This means that a primary way such predictions might err is if this trend in computing prices were to leave its long run trajectory. This must presumably happen eventually, and has purportedly happened with other exponential trends in information technology recently.1
This page outlines our assessment of whether the long run trend is on track very recently, as of late 2017. This differs from assessing the long run trend (as we do here) in that it requires recent and relatively precise data. Data that may be off by one order of magnitude is still useful when assessing a long run trend that grows by many orders of magnitude. But if we are judging whether the last five years of that trend are on track, it is important to have more accurate figures.
Sources of evidence
We sought public data on computing performance, initial price, and date of release for different pieces of computing hardware. We tried to cover different types of computing hardware, and to prioritize finding large, consistent datasets using comparable metrics, rather than one-off measurements. We searched for computing performance measured using the Linpack benchmark, or something similar.
We ran into many difficulties finding consistently measured performance in FLOPS for different machines, as well as prices for those same machines. What data we could find used a variety of different benchmarks. Sometimes performance was reported as ‘FLOPS’ without explanation. Twice the ‘same’ benchmarks turned out to give substantially different answers at different times, at least for some machines, apparently due to the benchmarks being updated. Performance figures cited often refer to ‘theoretical peak performance’, which is calculated from the computer’s specifications, rather than measured, and is higher than actual performance.
Prices are also complicated, because each machine can have many sellers, and each price fluctuates over time. We tried to use the release price, the manufacturer’s ‘recommended customer price’, or similar where possible. However, many machines don’t seem to have readily available release prices.
These difficulties led to many errors and confusions, such that progress required running calculations, getting unbelievable results, and searching for an error that could have made them unbelievable. This process is likely to leave remaining errors at the end, and those errors are likely to be biased toward giving results that we find believable. We do not know of a good remedy for this, aside from welcoming further error-checking, and giving this warning.
Evidence
GPUs appear to be substantially cheaper than CPUs, cloud computing (including TPUs), or supercomputers.2 Since GPUs alone are at the frontier of price performance, we focus on them. We have two useful datasets: one of theoretical peak performance, gathered from Wikipedia, and one of empirical performance, from Passmark.
GPU theoretical peak performance
We collected data from several Wikipedia pages, supplemented with other sources for some dates and prices.3 We think all of the performance numbers are theoretical peak performance, generally calculated from specifications given by the developer, but we have not checked Wikipedia’s sources or calculations thoroughly. Our impression is that the prices given are recommended prices at launch, by the developers of the hardware, though again we have only checked a few of them.
We look at Nvidia and AMD GPUs and Xeon Phi processors here because they are the machines for which we could find data on Wikipedia easily. However, Nvidia and AMD are the leading producers of GPUs, so this should cover the popular machines. We excluded many machines because they did not have prices listed.
Figure 1 shows performance (single precision) over time for processors for which we could find all of the requisite data.
The recent rate of progress in this figure looks like somewhere between half an order of magnitude in the past eight years and an order of magnitude in the past ten, for an order of magnitude about every 10-16 years. We don’t think the figure shows particular slowing down—the most cost-effective hardware has not improved in almost a year, but that is usual in the rest of the figure.
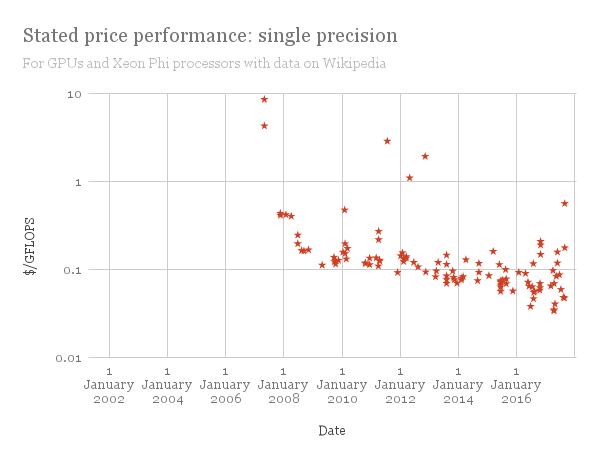
We also collected double precision performance figures for these machines, but the machines do not appear to be optimized for double precision performance,4 so we focus on single precision.
Peak theoretical performance is generally higher than actual performance, but our impression is that this should be by a roughly constant factor across time, so not make a difference to the trend.
GPU Passmark value
Passmark maintains a collection of benchmark results online, for both CPUs and GPUs. They also collect prices, and calculate price for performance (though it was not clear to us on brief inspection where their prices come from). Their performance measure is from their own benchmark, which we do not know a lot about. This makes their absolute prices hard to compare to others using more common measures, but the trend in progress should be more comparable.
We used archive.org to collect old versions of Passmark’s page of the most cost-effective GPUs available, to get a history of price for passmark performance. The prices are from the time of the archive, not necessarily from when the hardware was new. That is, if we collected all of the results on the page on January 1, 2013, it might contain hardware that was built in 2010 and has maybe come down in price due to being old. You might wonder whether this means we are just getting a lot of really cheap old hardware with hardly any performance, which might be bad in other ways and so not represent a realistic price of hardware. This is possible, however given that people show interest in this (for instance, Passmark keep these records) it would be surprising to us if this metric mostly caught useless hardware.
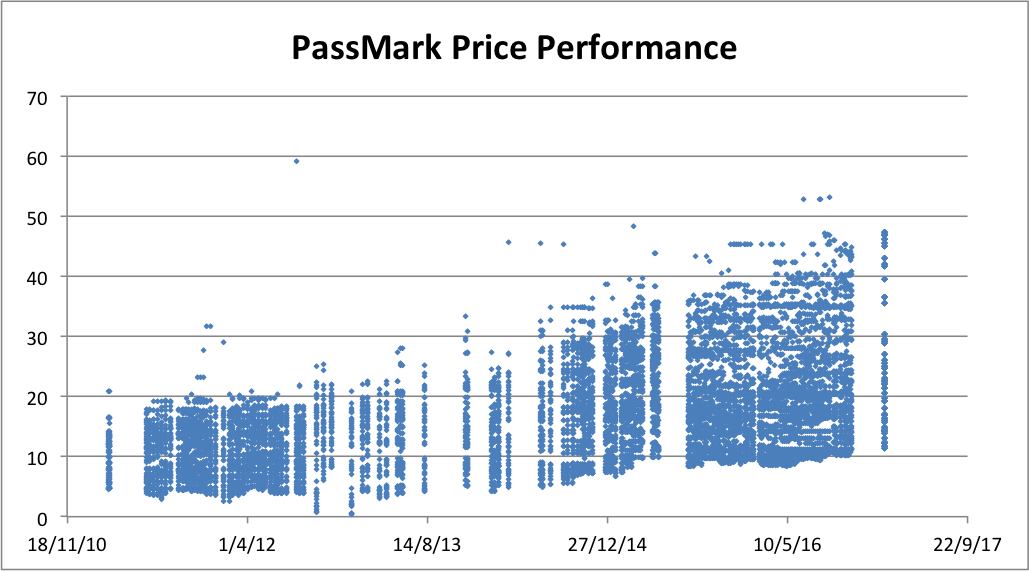
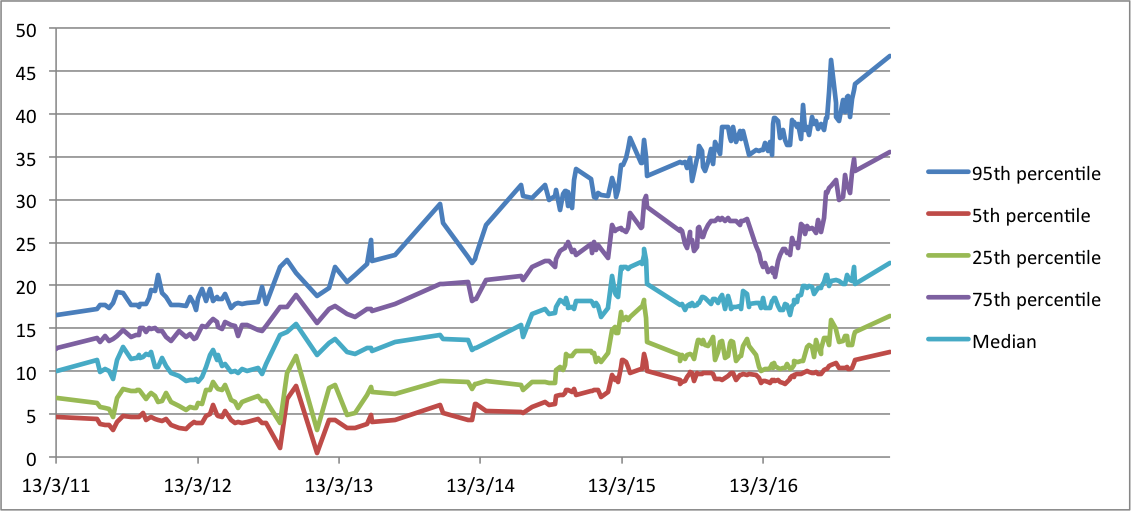
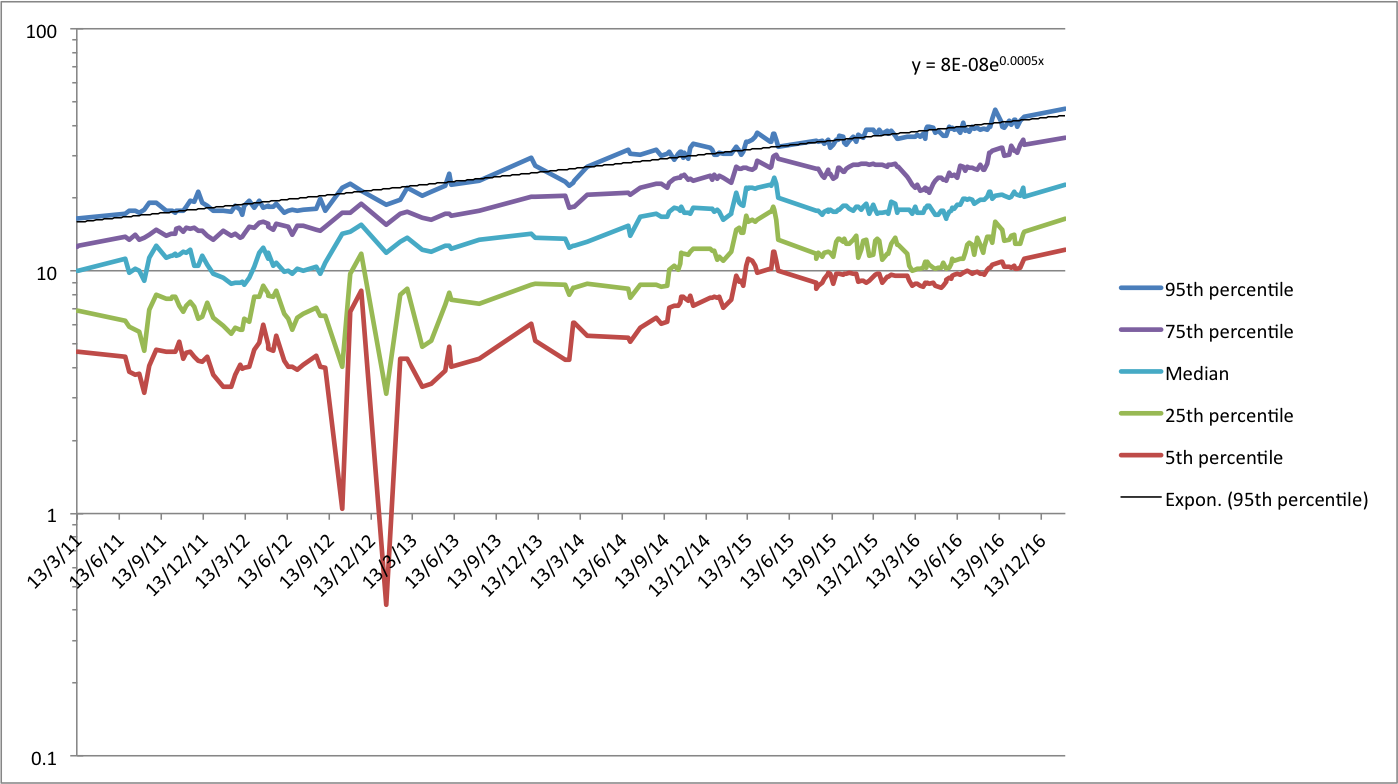
We are broadly interested in the cheapest hardware available, but we probably don’t want to look at the very cheapest in data like this, because it seems likely to be due to error or other meaningless exploitation of the particular metric.5 The 95th percentile machines (out of the top 50) appear to be relatively stable, so are probably close to the cheapest hardware without catching too many outliers. For this reason, we take them as a proxy for the cheapest hardware.
Figure 4 shows the 95th percentile fits an exponential trendline quite well, with a doubling time of 3.7 years, for an order of magnitude every 12 years. This has been fairly consistent, and shows no sign of slowing by early 2017. This supports the 10-16 year time we estimated from the Wikipedia theoretical performance above.
Other sources we investigated, but did not find relevant
- The Wikipedia page on FLOPS contains a history of GFLOPS over time. The recent datapoints appear to overlap with the theoretical performance figures we have already.
- Google has developed Tensor Processing Units (TPUs) that specialize in computation for machine learning. Based on information from Google, we estimate that they perform around 1.05 GFLOPS/$.
- In 2015, cloud computing appeared to be around a hundred times more expensive than other forms of computing.6 Since then the price appears to have roughly halved.7 So cloud computing is not a competitive way to buy FLOPS all else equal, and the price of FLOPS may be a small influence on the cloud-computing price trend, making the trend less relevant to this investigation.
- Top supercomputers perform at around $3/GFLOPS, so they do not appear to be on the forefront of cheap performance. See Price performance trend in top supercomputers for more details.
- Geekbench has empirical performance numbers for many systems, but their latest version does not seem to have anything for GPUs. We looked at a small number of popular CPUs on Geekbench from the past five years, and found the cheapest to be around $0.71/GFLOPS. However there appear to be 5x disparities between different versions of Geekbench, which makes it less useful for fine-grained estimates.
Conclusions
We have seen that the theoretical peak single-precision performance of GPUs is improving at about an order of magnitude every 10-16 years. And that the Passmark performance/$ trend is improving by an order of magnitude every 12 years. These are slower than the long run price-performance trends of an order of magnitude every eight years (75 year trend) or four years (25 year trend).
The longer run trends are based on a slightly different set of measures, which might explain a difference in rates of progress.
Within these datasets the pace of progress does not appear to be slower in recent years relative to earlier ones.
- For instance processor clock speed and traditional Moore’s law
- See ‘Other sources…’ below.
- Wikipedia pages: Xeon Phi, List of Nvidia Graphics Processing Units, List of AMD Graphics Processing Units
Other sources are visible in the last column of our dataset (see ‘Wikipedia GeForce, Radeon, Phi simplified’ sheet)
- Performance is usually measured either as ‘single precision’ or ‘double precision’. Roughly, the latter involves computations with numbers that are twice as large, in the sense of requiring twice as many bits of information to store them (see here and here). The trend of double precision performance from this dataset saw no progress in five years, and in fact became markedly worse (see figure below).
Our understanding is that GPUs are generally not optimized for double precision performance, because it is less relevant to the applications that they are used for. Many processors we looked at either purportedly did not do double precision operations at all, or were up to thirty-two times slower at them. So our guess is that we are seeing movement toward sacrificing double precision performance for single precision performance, rather than a slowdown in double precision performance where it is intended. So we disregard this data in understanding the overall trend.
- One reason it seems unlikely to us that the very cheapest numbers are ‘real’ is because they are quite noisy (see figure 2)—high numbers appear and disappear, whereas if we had made real progress in hardware technology, we might expect cheap hardware available in 2012 to also be available in 2013. Another reason is that in similar CPU data that we scraped from Passmark, there is a clear dropoff in high numbers at one point, which we think corresponds to slightly changing the benchmark to better measure those machines.
- See Current FLOPS Prices, or 2015 FLOPS prices.
- As of October 5th, 2017, renting a c4.8xlarge instance costs $0.621 per hour (if you purchase it for three years, and pay upfront) When we last checked this in around April 2015, the price for the same arrangement was $1.17 / hour.
Google released TPU to the public today (in beta):
https://cloudplatform.googleblog.com/2018/02/Cloud-TPU-machine-learning-accelerators-now-available-in-beta.html
“Built with four custom ASICs, each Cloud TPU packs up to 180 teraflops of floating-point performance”
“usage is billed by the second at the rate of $6.50 USD / Cloud TPU / hour.”
Someone might ask why this is so many orders of magnitude cheaper than Katja’s report: My understanding (I am not an expert): The reason is data types. I think Google is reporting metrics based on their custom datatype ‘quantization’ which is similar to 8-bit minifloat:
https://www.tensorflow.org/performance/quantization
Personally I would like to defend Google here: if their data type works for machine learning, why not report FLOPS based on that data type?
With regard to this article, I haven’t put in any time to determining if Katja used only double precision floating point or whether the hardware vendors (like Nvidia) even report on lower precision performance.
Sorry for being wrong+lazy+sloppy
AIImpacts article today is correct https://aiimpacts.org/2018-price-of-performance-by-tensor-processing-units/
I jumped to conclusions. To quote vomjom from hackernews
“TPUv1, which is built for quantized 8-bit inference. The TPUv2, which was announced in this blog post, is for general purpose training and uses 32-bit weights, activations, and gradients.
It will have very different performance characteristics.”
Google describes TPUv1 high performance as 15x to 30x faster at the following link (but tpuv1 is not available to the public) https://cloudplatform.googleblog.com/2017/04/quantifying-the-performance-of-the-TPU-our-first-machine-learning-chip.html
No worries, and thanks for pointing out the blog post with price figures in it to us. I remain confused, since TPUs seem to be considered to be much better than other things. I guess this is something to do with the cloud price being high relative to the internal price (in general cloud prices seem to be extremely high compared to the price of buying hardware), but I don’t fully understand why that would be.
Thanks a lot for sharing this with all of us you really know what you’re
talking about! Bookmarked. Kindly also seek advice
from my website =). We could have a hyperlink alternate arrangement between us