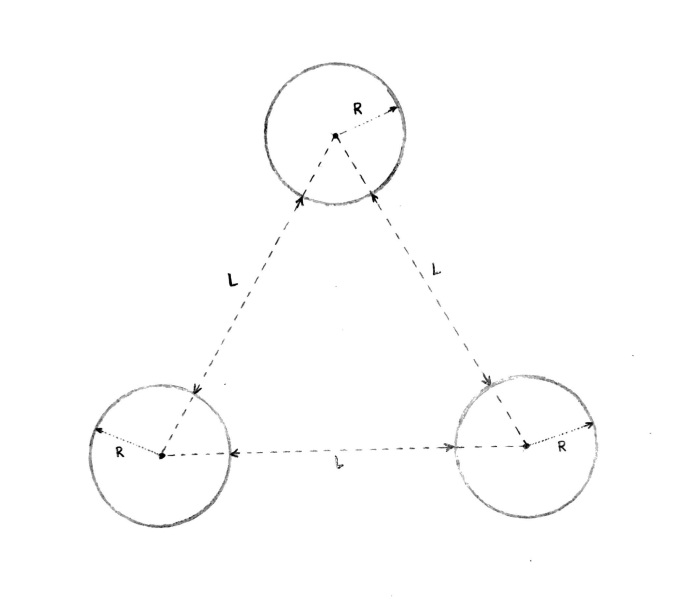
You Can’t Predict a Game of Pinball
The uncertainty in the location of the pinball grows by a factor of about 5 every time the ball collides with one of the disks. After 12 bounces, an initial uncertainty in position the size of an atom grows to be as large as the disks themselves. Since you cannot measure the location of a pinball with more than atom-scale precision, it is in principle impossible to predict the motion of a pinball as it bounces between the disks for more than 12 bounces.
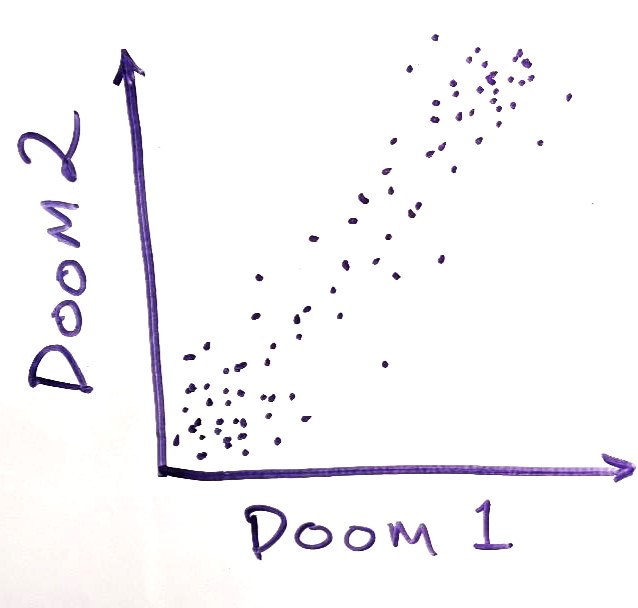
Against a General Factor of Doom
If you ask people a bunch of specific doomy questions, and their answers are suspiciously correlated, they might be expressing their p(Doom) for each question instead of answering the questions individually. Using a general factor of doom is unlikely to be an accurate depiction of reality. The future is likely to be surprisingly doomy in some ways and surprisingly tractable in others.
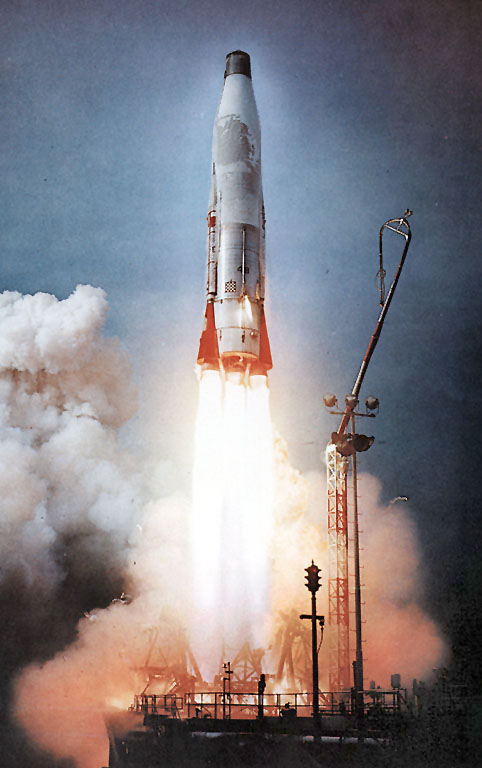
Discontinuous progress in history: an update
Katja Grace April 2020
We’ve been looking for historic cases of discontinuously fast technological progress, to help with reasoning about the likelihood and consequences of abrupt progress in AI capabilities. We recently finished expanding this investigation to 37 technological trends. This blog post is a quick update on our findings. See the main page on the research and its outgoing links for more details.
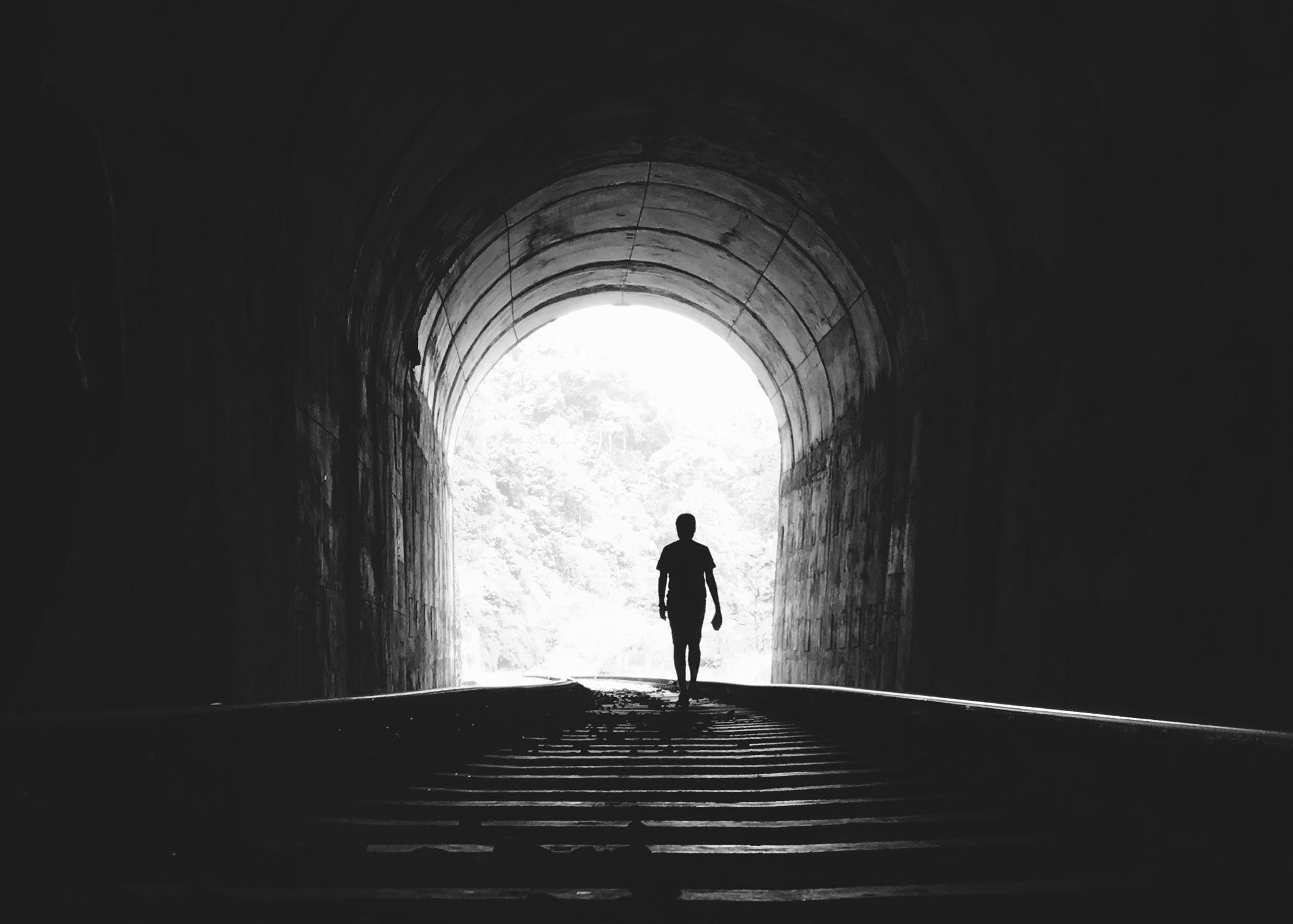
Takeaways from safety by default interviews
Asya Bergal
Last year, several researchers at AI Impacts (primarily Robert Long and I) interviewed prominent researchers inside and outside of the AI safety field who are relatively optimistic about advanced AI being developed safely. These interviews were originally intended to focus narrowly on reasons for optimism, but we ended up covering a variety of topics, including AGI timelines, the likelihood of current techniques leading to AGI, and what the right things to do in AI safety are right now. (…)
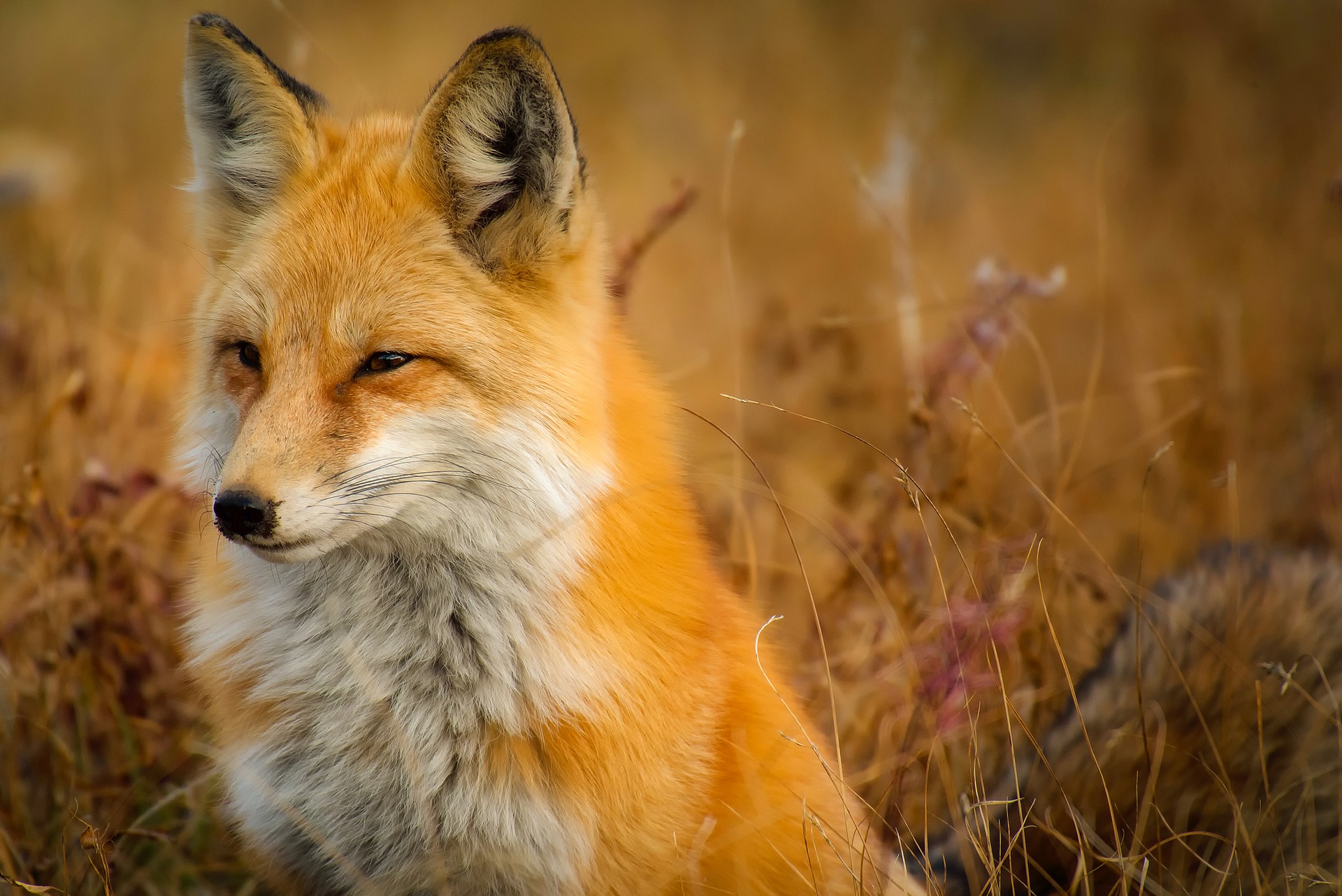
4 Trackbacks / Pingbacks
Comments are closed.